AI Powered Digital Newspaper – Feasibility and Implications
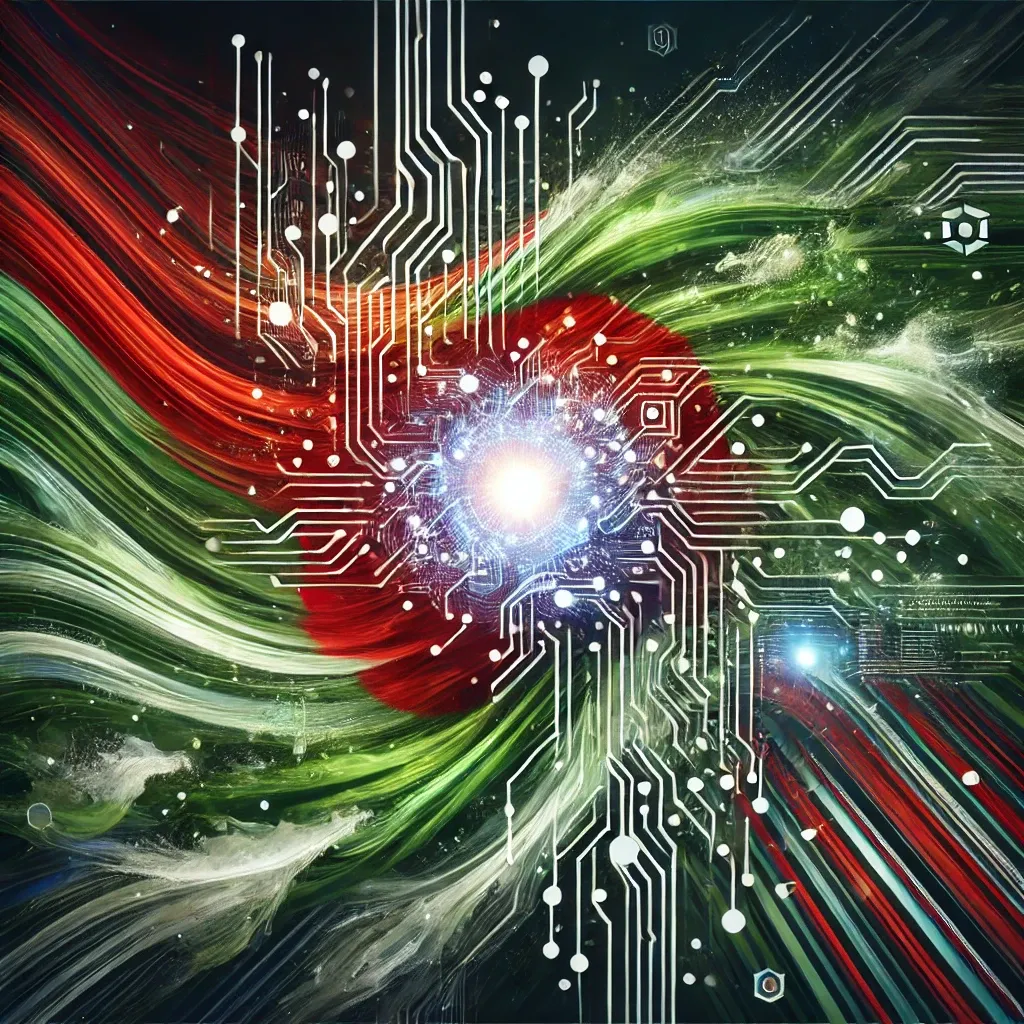
We are conducting a deep analysis of a new concept for a Bangladesh Digital Newspaper moderated entirely by AI, focusing solely on positive news and driven by democratic voting. This report will cover feasibility, ethical implications, technical implementation, economic viability, legal risks, and comparisons with existing models. It will also examine regulatory concerns, potential AI bias, scalability, and monetization strategies.
Feasibility Analysis
(How Documented uses WhatsApp to reach local immigrant communities - American Press Institute) (Welcome to Agrilife24 - Citizen Journalism in Bangladesh: Empowering Voices, Challenging Narratives)The proposed model leverages widespread WhatsApp usage (over 2 billion users globally, including 130+ million internet users in Bangladesh) as a channel for citizen news reporting. Technically, it is feasible to integrate WhatsApp through its Business API or chatbot frameworks, allowing users to submit text, images, and video clips as news content. For example, a fact-checking initiative in Africa is developing a WhatsApp chatbot for users to submit content for rapid AI verification (Ten fact-checking organisation receive grant from IFCN), demonstrating that user-generated content can be ingested and processed automatically. Likewise, news organizations have used WhatsApp to engage communities and gather tips, showing that real-time news inputs from citizens via messaging apps are practicable (How Documented uses WhatsApp to reach local immigrant communities - American Press Institute).
Building the backend requires an AI pipeline to filter and classify submissions. A natural language processing (NLP) model (trained on Bengali and English content) would assess the sentiment and subject of each submission to decide if it qualifies as “positive news about Bangladesh.” This could involve sentiment analysis (to gauge positive vs. negative tone) and entity recognition (to ensure the content relates to Bangladesh). Developing a robust classifier is doable – researchers have already created models that classify Bangla text sentiment into positive/negative categories (eftekhar-hossain/Bangla-News-Comments: Sentiment Analysis of ...). Images and videos would need computer vision algorithms to detect inappropriate or negative elements (e.g. violence or disaster imagery). If the AI flags content as not meeting the positivity criteria, it can automatically withhold or reject that submission. The AI would then autonomously publish the approved posts on a digital platform (website or app) and possibly redistribute them through WhatsApp broadcasts or social media. Distribution and personalization can be handled by AI recommendation systems, which many news outlets already use to tailor content to user preferences (What Percentage of News Articles Are AI Generated?).
Logistically, verifying user inputs and maintaining quality is a challenge but not insurmountable. Tying each submission to a phone number (WhatsApp account) inherently provides a layer of verification – one person per number – and discourages complete anonymity. Still, additional verification (like one-time passwords or registration) might be needed to prevent spam or duplicate accounts. The daily voting mechanism (one vote per phone number at $1 each) to define “positive news” would require a secure payment integration, perhaps via mobile money popular in Bangladesh (e.g. bKash) or carrier billing. Technically, collecting micropayments of $1 is feasible, but ensuring fairness (one vote per user) is crucial; mechanisms to detect and block multiple SIM use or other gaming of the vote would be needed. The AI system would also need to update its moderation parameters daily based on the user-voted definition. This could be done by treating the user vote outcome as a high-level directive or keyword list for that day – for instance, if voters decide “positive news” means stories of community improvement, the AI could prioritize and allow content tagged with community and development topics.
One concern is scalability of moderation. The volume of submissions could be high if the platform gains popularity, but AI moderation is designed to scale more efficiently than human editors. Major news wires already automate large portions of content production (the Associated Press generates ~40,000 of its 730,000 news articles per year using AI – about 5.5% of output (What Percentage of News Articles Are AI Generated?)). This speed and automation can free human staff from reviewing every post. However, fully automated platforms have to manage accuracy. Past experiences with citizen-reporting platforms show that much user-submitted content can be misleading or false if unvetted – for instance, the “Citizen” news app allows anyone to post local reports, and observers noted that less than 30% of those reports might be accurate (Artificial Intelligence and ‘Citizen Journalism’ Present Immediate and Present Danger to Media | Barrett Media). To address this, the AI could incorporate basic fact-checking (cross-referencing known news or Wikipedia data) for factual claims, and perhaps a human-in-the-loop for borderline cases. Nonetheless, from a pure feasibility standpoint, the combination of WhatsApp for intake and advanced AI for moderation and publishing is achievable with today’s technology. The core components (messaging APIs, NLP sentiment models, image recognition, automated publishing systems) already exist or are in active use by media and fact-checking organizations (Ten fact-checking organisation receive grant from IFCN) (How Documented uses WhatsApp to reach local immigrant communities - American Press Institute). With sufficient development effort and computing resources, an AI-run “positive news” newspaper in Bangladesh is technically within reach.
Ethical Considerations
Implementing an AI-driven news outlet that only publishes “positive news” raises significant ethical questions. Chief among these is the risk of censorship and distortion of reality. By design, negative news or critical reporting would be filtered out – effectively creating a news source with a strong positivity bias. This approach conflicts with journalism’s watchdog role of holding power to account by reporting problems and wrongdoing (News and the Negativity Bias: What the Research Says | by Christopher Reeve | The Whole Story). Ethically, who gets to decide what counts as “positive” is problematic. In this model, that definition is crowdsourced via daily votes, but as one media commentator noted in the context of AI-curated content: “Who is to determine what the good stuff is? We have already seen suppression of information” when AI selects only certain content (Artificial Intelligence and ‘Citizen Journalism’ Present Immediate and Present Danger to Media | Barrett Media). If the majority of users vote a narrow definition of “positive news” (for example, only praising government projects), the platform could become an echo chamber that omits any important news that is perceived as negative, even if it’s true and relevant. This selective coverage could amount to a form of algorithmic censorship, albeit driven by user preferences. It might also reinforce a “filter bubble,” where the audience is only exposed to feel-good stories and remains unaware of serious issues (poverty, corruption, disasters) that require attention.
There is also the ethical dimension of truth versus cheerfulness. While focusing on positive developments can inspire hope, doing so exclusively risks misleading the public about the state of the country. Citizens might get a falsely rosy picture if all they read are success stories. In extreme cases, this veers into propaganda territory – akin to state media that highlight only achievements and ignore failures. In Bangladesh’s context, this concern is acute because the government has in recent years cracked down on critical voices (using laws like the Digital Security Act) and often demands a positive portrayal of the nation (Bangladesh: New Digital Security Act is attack on freedom of expression - Amnesty International). A platform that “only publishes positive news about Bangladesh” could be seen as tacitly aligning with those censorship pressures, even if it’s privately run. There’s a thin line between promoting optimism and silencing criticism. Ethically, the platform would need to be transparent that it is a supplement to, not a substitute for, comprehensive news – otherwise it could be accused of propagandizing by omission.
On the other hand, there are some positive ethical arguments for this concept. The traditional news media’s negativity bias – “if it bleeds, it leads” – has been criticized for overwhelming audiences with despair and cynicism (Constructive Journalism | World's Best News) (Constructive Journalism | World's Best News). Studies show that constant negative news can cause people to disengage from news altogether out of stress or hopelessness, and can skew public perception to be more pessimistic than reality (Constructive Journalism | World's Best News) (Constructive Journalism | World's Best News). In contrast, highlighting positive news and solutions can empower and engage people rather than leaving them feeling helpless (News and the Negativity Bias: What the Research Says | by Christopher Reeve | The Whole Story). A dedicated positive-news outlet could fill a gap by reporting constructive stories that mainstream media underreport. Ethically, this contributes to a more balanced informational ecosystem – providing “a more contextualized picture of the world, without overemphasizing the negative” (Constructive Journalism | World's Best News). It aligns with the idea of constructive journalism, which aims to present news in a way that includes progress and solutions, not just problems, thereby giving audiences a sense of agency and inspiration. From this perspective, the platform could have social value by celebrating achievements, fostering national pride, and motivating readers (for instance, a story about a successful local social project might inspire others to replicate it).
Another ethical consideration is the voting mechanism for defining positive news. Charging $1 per vote and allowing the community to set the editorial tone is a novel democratic approach, but it introduces questions of equity and integrity. At one vote per phone number, theoretically every person has equal say for a small fee, but in practice, wealthier or more organized groups could mobilize many voters (or many SIM cards) to sway the definition. This could lead to a tyranny of the majority in content moderation – silencing minority viewpoints on what “positive” means. For example, human-rights advocates might consider a critical exposé leading to reforms as a positive outcome, but a majority of voters might define that as “too negative” and thus exclude it. Furthermore, monetizing votes might be seen as putting editorial policy up for sale. Ethically, news values should ideally not be determined by who pays, even if the fee is small. There’s a risk of undermining trust if users feel the definition of news can be bought (even though $1 is minor, it could accumulate or be subsidized by interested parties). On the flip side, the pay-to-vote system could discourage trolling and frivolous votes (since there’s a cost), potentially resulting in more thoughtful input from those who do vote.
Finally, AI-driven moderation brings its own ethical challenges. Removing human editors from the loop means decisions about publication are made by algorithms that lack human judgment and empathy. AI might not understand satire, context, or the cultural nuance behind a story, leading to unfair blocking of content. For instance, an AI could reject a news piece about a protest because it contains “negative” keywords, even if the overall angle is positive change. Without a human to override, contributors might have no recourse – raising issues of accountability and fairness in how their submissions are handled. Transparency in the AI’s decision criteria will be crucial to maintain user trust. Overall, the concept walks an ethical tightrope between uplifting readers and potentially misinforming them by omission. It will require careful governance (perhaps an ethics board or oversight committee) to ensure that “positive news” doesn’t simply become a glossy façade that hides reality. Balancing optimism with honesty will be key to preserving credibility.
Technical Implementation (AI Moderation, Verification, Content Generation)
(A group of white robots sitting on top of laptops photo – Free Computer Image on Unsplash) Advances in AI now enable algorithmic “newsrooms” capable of writing and curating content, though oversight and accuracy remain challenges (What Percentage of News Articles Are AI Generated?) (What Percentage of News Articles Are AI Generated?).
AI Content Moderation: The heart of this platform is an AI moderator that enforces the “positive news only” directive. This would likely be a multi-layered AI system. The first layer is a content filter powered by NLP models which screens incoming submissions (text or transcribed speech from videos) for sentiment and topic. One approach is to use a sentiment analysis model fine-tuned on Bangladeshi news content to identify whether a submission’s tone is positive, neutral, or negative. A simple sentiment score, however, may not be sufficient – the directive is not just about tone but also about relevance to Bangladesh and some notion of constructiveness. Therefore, a custom classification model could be trained with examples of what the community deems “positive news.” Over time, as daily votes provide feedback (essentially labels for content that was considered positive vs not), the model can learn patterns. Modern transformer-based models (like multilingual BERT or XLM-RoBERTa) have been successfully used for Bengali text classification (Sentiment Analysis For Bengali News Text Using LSTM - Medium), which suggests feasibility for this task. The model might check for certain disallowed content too (e.g., political criticism, crime reports – anything routinely considered “negative” under the evolving definition). If an item fails the positivity test, the AI drops it or perhaps queues it for review if a secondary check is desired.
For images and videos, computer vision techniques are needed. An AI vision model could perform object and scene recognition to flag graphic violence, protests, or other imagery likely associated with negative news. Additionally, image captioning or OCR (optical character recognition) could extract text from an image (like reading a poster in a photo) to ensure nothing in the image’s content violates the positivity rule. For example, an image with visible destruction (like a burned building) would be flagged as negative context. Likewise, a video might be analyzed via speech-to-text transcription of its audio and detection of distressing visuals. These AI models (for vision and audio) would work in tandem with the text classifier.
User Input Verification: Ensuring each news submission comes from a real, unique user is important for both trust and the one-vote-per-user system. WhatsApp itself verifies users by phone number, so simply using WhatsApp as the intake ensures a basic level of authenticity (it’s harder for bots to mass-create WhatsApp accounts compared to email, due to phone number verification). The platform could maintain a database of trusted contributors over time, perhaps giving frequent submitters a “verified citizen reporter” badge. To prevent abuse, rate limiting could be implemented (e.g., a single user can only submit a certain number of stories per day) and known spam content could be filtered by AI (many spam/advertising messages have detectable patterns that AI can learn). If needed, the system might integrate an ID verification for voters or contributors for an added layer (though that raises privacy issues). The daily voting process itself would use the phone number as the unique key; integrating a mobile payment API would allow the system to charge $1 and record the vote tied to that number. This process is similar to how some online contests or polls allow one vote per SMS or phone – technically straightforward. The main challenge is handling payments at scale securely (to avoid fraud in transactions), but partnering with local mobile payment services could offload that complexity.
AI Content Creation: Once content passes the positivity filter, the AI can also assist in generating and formatting the news article. Not all user submissions will be well-written or complete. An AI writing assistant (likely a large language model) can expand a short blurb into a full news story, correct grammar, and ensure a consistently upbeat tone. For instance, if a user submits “Local youth cleaned up the neighborhood lake, great success,” the AI could flesh this out into a short article: headline, a few paragraphs describing who organized the cleanup, quotes (if provided or fabricated carefully), and the positive impact on the community. Caution is needed here: AI generation should stick to facts provided to avoid hallucination of details. One approach is to use template-based generation for common story types. The Associated Press and other outlets have used automated templates for things like sports scores or earnings reports for years (The AP announces five AI tools to help local newsrooms with tasks ...). The AI could have predefined structures for, say, “Local Community Improvement” stories or “Inspiring Individual Achievement” stories, into which it plugs the specifics from the user’s submission.
A modern generative model like GPT-4 (or an open-source equivalent fine-tuned on journalism) could be employed to rewrite content in a polished, engaging style. It could also translate content (e.g., take a Bengali submission and produce an English version or vice versa) to reach a wider audience. The directive of positivity can be enforced at the generation stage too: the AI writer can be instructed via prompt or fine-tuning to maintain an optimistic framing. For example, if summarizing an event that has mixed outcomes, the AI would be guided to emphasize the beneficial aspects. However, editorial judgment is tricky to encode – the AI might need periodic human feedback to ensure it’s not inadvertently spinning or exaggerating facts to sound positive.
Distribution & Personalization: Once generated, content can be published on a digital platform. The AI can also handle scheduling and distribution: posting articles to a website, sending out a daily digest via WhatsApp or email, and sharing on social media. AI-driven recommendation engines (similar to those used by large news sites) could personalize the user experience – for instance, a reader who often clicks on tech success stories might be automatically shown more of those. This increases engagement and is technically implemented via predictive modeling on user behavior (What Percentage of News Articles Are AI Generated?). Given the niche (positive Bangladeshi news), even a basic chronological feed might suffice, but personalization could be a value-add.
Continuous Learning and Moderation: Over time, the AI can improve its moderation precision by learning from what gets upvoted or downvoted. Each day’s user vote on the definition of positive news provides a feedback loop. If, for example, users vote that they want “economy and development” positive news today, the AI could adjust weights to favor economic success stories. This dynamic tuning might be achieved by having a set of thematic classifiers or filters that can be toggled on/off based on the vote result. While dynamic retraining of an NLP model daily is likely too slow, the system could have pre-trained categories (economic, cultural, environmental, etc.) and then apply the chosen category’s filter rules for that day. This modular approach is more engineering than AI research – essentially, encode a few possible “modes” for the AI (one day focus on, say, national pride stories, another on local community stories, etc., as defined by prior input from editors or user trials). The vote then selects the mode.
From a resource standpoint, implementing these AI components would require a solid infrastructure: cloud servers with GPU/TPU for model inference (especially if handling images and large language models). Latency must be low enough that when a user submits something, the decision to publish or reject happens in perhaps seconds. Given current technology, this is achievable – content moderation algorithms on social networks already operate at huge scales in near-real-time (Benefits & Challenges of Using AI for Content Moderation). The key is ensuring accuracy, which leads to the next point: even the best AI will make mistakes (false positives or negatives). Therefore, a fail-safe could be to have human moderators or editors in a limited role: for example, review a random sample of AI-approved stories to ensure quality, or handle user appeals if their submission was wrongly rejected. This hybrid approach would combine AI efficiency with a human layer for accountability (What Percentage of News Articles Are AI Generated?). Overall, the technical implementation involves orchestrating multiple AI subsystems (for text, vision, generation, personalization) and integrating them with the user-facing WhatsApp interface and web platform. All of these components exist in some form today, making the implementation challenging but feasible with a dedicated development effort.
Comparison with Existing Models (Case Studies Worldwide)
Although the exact concept – a WhatsApp-based, AI-run, positive-only newspaper – is novel, it draws on trends and examples from around the world. There are precedents in both the content approach (positive news) and the participatory model (citizen-sourced journalism moderated by a platform). Examining these can shed light on potential outcomes:
- “Good News” Media Sites: There is a growing niche of news outlets devoted entirely to positive or uplifting stories. For example, the Good News Network (GNN), founded in 1997, has compiled over 21,000 positive news stories to date and serves as a “daily dose of hope” for millions of readers (About GNN - Good News Network). GNN curates inspiring articles from around the world and has proven that a sustained appetite for good news exists; it even offers an app and newsletter to distribute these stories widely (About GNN - Good News Network). Similarly, Positive News (UK) is a publication that bills itself as “the magazine for good journalism about what’s going right” (Good news only: Where to find good news online). Founded in 1993, Positive News transitioned from a print quarterly to a digital-first outlet, focusing on constructive journalism and solutions-oriented reporting (Positive News - Wikipedia). Notably, Positive News is structured as a cooperative owned by readers – over 1,500 readers invested in it via a crowdfunding campaign, raising £260,000 to support its mission (Positive News - Wikipedia). This illustrates that communities will financially support media that aligns with their values (akin to how our model asks the community to pay $1 votes to shape content).
(Good news only: Where to find good news online) Niche outlets like the “Goodnewspaper” focus exclusively on uplifting stories, reflecting a public desire for positive media (Good news only: Where to find good news online).
The existence of these outlets demonstrates viability for positive-only content: they have attracted audiences and funding. Our Bangladesh-focused model could be seen as a localized, more interactive extension of this idea. However, GNN and Positive News still employ human editors to select and write stories; they don’t allow anyone to post content freely. This is where our concept diverges by embracing citizen contributions on a large scale.
- Citizen Journalism Platforms: The idea of a news site powered by user contributions has been tried in various forms. A landmark example is OhmyNews in South Korea, launched in 2000 with the motto “Every citizen is a reporter.” OhmyNews became one of the most successful citizen journalism efforts globally (A Visit to OhmyNews | PR Watch). It amassed a network of over 60,000 citizen reporters who submitted stories, which were then vetted and edited by a professional staff of about 60 editors (A Visit to OhmyNews | PR Watch). At its peak, OhmyNews had huge influence and even an international edition. The success was attributed to blending grassroots contributions with editorial oversight – citizen reporters had to follow a code of ethics, and their work was fact-checked and curated. This ensured a level of quality and trust. In comparison, the Bangladesh digital newspaper would replace that human editorial layer with AI. The lesson from OhmyNews is that while citizen reporting can produce a vast range of stories, maintaining standards is crucial. If our AI moderation can achieve something analogous to what OhmyNews’ human editors did, the platform could flourish; if it cannot, the site might be flooded with low-quality or biased posts that undermine its credibility.
Another modern example is the “Citizen” mobile app (popular in some U.S. cities), where users post real-time alerts about local incidents (accidents, crimes, etc.). It relies on community reports and some algorithmic sorting, but minimal verification. As noted by a media analyst, Citizen app content can be wildly inaccurate – with everything from hoaxes (like a fake “pickle attack” report) to real incidents with wrong details being posted (Artificial Intelligence and ‘Citizen Journalism’ Present Immediate and Present Danger to Media | Barrett Media). This underlines the importance of moderation. Citizen app’s focus is actually mostly negative news (crime alerts), which is the opposite of our platform’s positivity focus, but the core challenge (user-generated reports) is similar. The Bangladesh platform can learn from this by incorporating verification steps that Citizen lacks. Interestingly, Citizen app does provide a lot of video footage and firsthand information to news outlets (who monitor it), showing that users are willing to be “eyes on the ground.” If we channel that willingness but for good news, we might tap into many unnoticed positive stories nationwide.
- Social Media & Voting Models: The concept of users voting on content has parallels in social media and online communities. Platforms like Reddit allow users to upvote posts, indirectly determining which content is most visible. Our model formalizes that by letting users vote on the criteria for content (and charging for it). While there isn’t a direct case of paying to set editorial policy daily, there are related ideas: for instance, some blockchain-based social networks like Steemit have tokens that users stake to promote content (essentially a paid voting mechanism for content ranking). Those platforms have shown that financially incentivized voting can engage users, but also that “whales” (big spenders) can dominate outcomes – a cautionary tale for fairness. Another partly similar initiative was Wikitribune (launched by Wikipedia’s Jimmy Wales in 2017), which aimed to combine professional journalists with volunteer contributors to produce factual news and fight fake news (Wikipedia's Jimmy Wales to set up global news website). Wikitribune didn’t restrict to positive news, but it explored community involvement in news production. It ultimately struggled and pivoted (becoming a more conventional discussion platform), suggesting that getting the community and professionals to jointly run a news site is challenging. In our case, replacing professionals with AI is an untested twist.
- Government or NGO “good news” initiatives: It’s worth noting that in some countries, especially where governments control media, the idea of promoting only positive news is often mandated. For example, state media in countries like China tend to emphasize “positive energy” stories and minimize negative press as a propaganda strategy (Pandemics & propaganda: How Chinese state media creates and ...). And in 2020s Bangladesh, state officials have sometimes expressed the desire for media to focus on nation-building news. While our proposed platform is independent, it might operate in a similar content space (i.e. mostly developmental success stories, human triumphs, etc.). There have been NGO-led news projects too, such as World’s Best News (originating in Denmark), which partners with organizations to publish positive stories about global development and the UN Sustainable Development Goals. World’s Best News practices constructive journalism and has had campaigns to distribute good news publications to the public to counter pessimism (Constructive Journalism | World's Best News) (Constructive Journalism | World's Best News). This shows that framing journalism around solutions and progress can gain support from civil society and even corporate sponsors.
In summary, the proposed Bangladesh digital newspaper marries two ideas with precedent: positive-only content (as seen in Good News Network, Positive News magazine, etc.) and citizen-sourced journalism (as seen in OhmyNews, user-driven apps, Reddit-style voting). Each component has success stories, but their combination, especially with AI moderation, is experimental. The case studies suggest a few recommendations: maintain content quality (learning from OhmyNews’ editorial process), ensure verification to avoid the pitfalls of unmoderated user reports (learning from Citizen app’s issues), and engage the community for support (as Positive News did via co-ownership, which is analogous to users paying and voting in our model). If executed well, the platform could become a showcase for how technology and community can create an upbeat alternative to mainstream news.
Regulatory & Legal Compliance in Bangladesh
Operating a news platform in Bangladesh requires careful navigation of media laws and regulations. Bangladesh has relatively strict controls on digital content and journalism, especially since the passage of the 2018 Digital Security Act (DSA). This law has broad and vaguely defined provisions that criminalize online content which may disturb the “law and order,” hurt religious sentiments, or criticise the nation’s founding principles (Bangladesh: New Digital Security Act is attack on freedom of expression - Amnesty International) (Bangladesh: New Digital Security Act is attack on freedom of expression - Amnesty International). In practice, the DSA has been used as a tool to crack down on journalists, activists, and ordinary citizens for social media posts critical of the government (How Bangladesh’s Digital Security Act Is Creating a Culture of Fear | Carnegie Endowment for International Peace). For instance, a prominent writer died in custody after being detained under DSA for Facebook posts, and teenage students have been arrested for simply sharing content deemed objectionable (How Bangladesh’s Digital Security Act Is Creating a Culture of Fear | Carnegie Endowment for International Peace). The environment is such that fear of legal repercussions has led to widespread self-censorship among netizens (Welcome to Agrilife24 - Citizen Journalism in Bangladesh: Empowering Voices, Challenging Narratives).
Given this context, a “positive news only” platform might actually find itself in a favorable position with authorities, since by design it avoids critical or negative journalism that could trigger DSA clauses. Content that is complimentary of Bangladesh or highlights successes is unlikely to violate provisions about defamation or “anti-state propaganda.” In fact, Section 21 of the DSA punishes “propaganda against the spirit of the Liberation War, Father of the Nation, national anthem or flag” with life imprisonment (Bangladesh: New Digital Security Act is attack on freedom of expression - Amnesty International). A site that scrupulously publishes only positive stories would almost inherently steer clear of such offenses. Likewise, Section 25 punishes statements that could “hurt religious values or sentiments” (Bangladesh: New Digital Security Act is attack on freedom of expression - Amnesty International) – by focusing on positive angles, the platform would likely avoid edgy or critical discussions of sensitive topics. In a sense, the concept aligns with what the government often urges media to do: project a positive image of the country. This could reduce the risk of direct legal action against the platform for its content.
However, there are still regulatory hurdles and risks. First, Bangladesh requires news websites to register with the government. In 2020, the Ministry of Information started mandating that all online news portals obtain official registration, and by 2021 they announced that new online news sites must be approved before launch (Bangladesh to introduce pre-registration rules for news websites in 2022 ) (Bangladesh to introduce pre-registration rules for news websites in 2022 ). This is ostensibly to ensure adherence to “professional journalism” standards. Our digital newspaper, even if AI-run, would need to go through this registration process to be legal. That involves submitting an application detailing the ownership, editorial responsibility, and other information. A potential complication is that the concept doesn’t have a traditional editor (the AI is the “editor”). Bangladeshi law may require a named editor or publisher who can be held accountable. For compliance, the platform’s owners might have to designate someone (perhaps the project founder or a supervising journalist) as the legally responsible Editor to satisfy regulators. Operating without registration could lead to being shut down or blocked by the Bangladesh Telecommunication Regulatory Commission (BTRC), as authorities have cracked down on unregistered news sites in the past (Bangladesh to introduce pre-registration rules for news websites in 2022 ) (Bangladesh to introduce pre-registration rules for news websites in 2022 ).
Another legal aspect is liability for user-generated content. If despite all precautions, a user manages to post something that the government deems offensive or fake, the platform could be held liable. The DSA allows police to arrest anyone involved in publishing offending digital content without a warrant (Bangladesh: New Digital Security Act is attack on freedom of expression - Amnesty International). This could include the platform administrators. Even if content is quickly removed by AI, the act of having it posted could pose risks if screenshots circulate. The platform would be wise to have clear terms of service that forbid any illegal content and to log moderation actions to show diligence in compliance. Fast removal mechanisms (in case a government agency flags something) should be in place to avoid escalation.
Furthermore, if the platform becomes influential, political sensitivities might arise. For example, which positive stories are highlighted might draw scrutiny. If user votes consistently favor stories of a certain political leaning (say, praising opposition-led local governments’ achievements), the ruling party might take issue despite the generally positive nature of the news. Government intervention could range from subtle (pressure to include more government successes as “positive news”) to overt (legal notices or censorship). The single-directive policy (“only positive news”) might protect against most direct confrontation, but it is not foolproof. There have been cases in Bangladesh of seemingly innocuous content creators getting in trouble due to misinterpretation or shifts in political winds.
It’s also important to consider laws beyond the DSA. Bangladesh has defamation laws and privacy laws that could be relevant. A user might submit a “positive” story about a person that that person doesn’t want published (violating privacy), or in celebrating one company’s success inadvertently make false claims about another. The platform must therefore moderate not just for positivity but also for libel, accuracy, and privacy. AI moderation would need to be attuned to these issues (for instance, not publishing personal details without consent in a feel-good human interest story).
Additionally, the platform should comply with any data localization or protection rules. If it stores user data (phone numbers, votes, etc.), Bangladeshi regulations (or upcoming ones under a new draft cybersecurity ordinance (GNI Statement on Recent Digital Regulations in Bangladesh)) might require certain handling of that data. As an emerging tech-driven media, it would also be on the radar of the BTRC which has broad powers to block online content deemed harmful (Bangladesh: New Digital Security Act is attack on freedom of expression - Amnesty International).
In summary, legally the concept is workable in Bangladesh if it adheres to registration requirements and remains strictly non-controversial. Its positive-only mandate could act as a shield against many of the speech restrictions that have entangled other media (Bangladesh: New Digital Security Act is attack on freedom of expression - Amnesty International). Nonetheless, it cannot ignore the formal regulations: getting a publishing license, appointing a responsible authority, and swiftly complying with takedown orders are all part of operating in Bangladesh’s media landscape. Proactively establishing a line of communication with regulators (perhaps assuring them that this platform is “nation-friendly”) could help. The paradox is that the very thing that raises ethical questions (censoring negative news) is what might keep the platform on the right side of Bangladeshi law. The owners will need to continuously monitor the legal climate and be ready to adapt policies if laws change (e.g., if a new law required even positive user content to undergo government screening, the platform would have to adjust).
Economic Viability and Sustainability
A critical question is whether this model can sustain itself financially. The revenue model suggested – users paying $1 per vote to decide the definition of positive news each day – is an innovative form of crowdfunding, but its sufficiency is uncertain. Let’s break down potential revenue and costs, and explore alternative income streams:
User Voting Revenue: If the platform manages to build a passionate user base, this could be a steady income. Suppose, optimistically, 10,000 users vote each day at $1 – that’s $10,000/day or about $3.65 million a year. In Bangladesh’s context, getting 10,000 daily paying users would be quite ambitious. More realistically, the voter pool might be in the hundreds or low thousands in early stages. The voting fee also functions as a sort of voluntary subscription – only those deeply engaged will pay regularly. It’s possible the vote revenue primarily covers certain costs (like server bills or marketing) but not all. However, even a smaller number of votes can help. The key is that this model crowdsources funding and editorial guidance simultaneously, akin to a community-funded news co-operative. The earlier example of Positive News (UK) raising funds from readers (Positive News - Wikipedia)bodes well – people do pay to support positive journalism. The platform could consider offering recognition or minor perks to frequent voters (to incentivize participation), essentially making them feel like members or stakeholders.
Advertising: Online advertising could be a major revenue source. Positive news is generally brand-safe content (no graphic violence, no divisive politics), which is very attractive to advertisers. Companies would likely be happy to have their ads appear next to uplifting stories about Bangladesh’s progress. Possible advertisers could include tourism boards (promoting Bangladesh’s positive image), local businesses wanting goodwill association, or multinational brands doing CSR campaigns. The platform can integrate banner ads, sponsored content (clearly labeled), or even short sponsor messages in the WhatsApp broadcasts. Given the large number of internet users in Bangladesh, if the site gains high traffic, ad revenue could be significant. One consideration: if the platform is framed as a sort of public-interest project, heavy advertising might clash with its ethos. But many “good news” sites do carry ads; Good News Network, for instance, sustains itself partly through ad impressions and also through donations/memberships (About GNN - Good News Network). In balancing user experience, the site could limit ad intrusion (maybe only a few high-quality sponsors rather than spammy ads). Additionally, because content is in both English and Bengali potentially, it might attract an international Bangladeshi diaspora audience – that widens the ad market (diaspora readers could be shown ads for remittance services, flights to Bangladesh, etc.).
Sponsorships and Partnerships: Beyond traditional ads, the platform might seek sponsorships. For example, a telecom company or bank might sponsor a section of the site (“This week’s positive news in tech is brought to you by…”) as part of their PR strategy. NGOs or development agencies could also partner to highlight success stories of projects – essentially paying to get their positive case studies featured (again, marked as sponsored). Caution is needed to keep editorial independence (the single directive should come from the community votes, not from sponsors’ agendas), but alignment is easier when everyone wants positive stories. If done transparently, sponsorship could provide a stable revenue. Companies love to associate with good news because it improves brand sentiment.
Premium Subscriptions: In addition to the voting mechanism, the platform could offer a premium tier for subscribers. For, say, a monthly fee, subscribers might get an ad-free experience, early access to stories, or exclusive positive reports (perhaps a weekly long-form inspirational story not available to free users). They could also get perks like the ability to see behind-the-scenes on how AI curates news, or even a limited number of free voting tokens. Since the content itself is positive (and presumably widely shareable), putting it fully behind a paywall may not be wise – reach would be more important. But a freemium model where casual readers see content free (with ads), and superfans support with a subscription, can work. Many online news outlets use this model. The trick is convincing users to pay for something that is also available for free. The unique selling point here is that by subscribing or voting, they aren’t just buying content – they are participating in a movement to spread positivity. That emotional appeal can drive voluntary payments (similar to how Wikipedia asks users for donations even though content is free, or how The Guardian’s readers contribute to keep it open-access).
Cost Structure: On the cost side, the platform may benefit from lower human resource expenses (since AI is doing moderation and content creation). But AI isn’t free – there are costs for cloud computing, software development, and maintenance. Initially, there will be development costs to build the system (engineers, AI specialists) which might require investment capital or grants. Ongoing costs include server hosting (especially if handling images/videos), API costs if using third-party AI services, and a small team to oversee operations (even if not for content, you’d have people managing the tech and community outreach). If the site grows, content volume and user interactions (comments, etc.) might require scaling the infrastructure. However, compared to a traditional news outlet with dozens of reporters and editors on payroll, this model could be lean.
Growth and Network Effects: The economic viability will improve as the user base grows. More contributors mean more content (which can attract more readers); more readers mean more potential voters and ad impressions. There’s a virtuous cycle if the platform catches on. To jumpstart this, some initial marketing is needed – possibly social media campaigns or partnerships with local organizations that promote the app/WhatsApp number for submitting stories. The platform could even cooperate with schools or universities, encouraging students to report good news from their communities, thereby seeding content early on. This community engagement strategy might require some expense (events, small rewards for best contributions, etc.), but it builds a loyal user base that is more likely to pay that $1 vote or subscribe out of affinity.
Alternate Funding: If user payments and ads are slow initially, the project could seek external funding to sustain itself. Options include grants (from media development organizations or tech for good funds) since the idea overlaps with civic tech, journalism innovation, and positive social impact. For example, an innovation fund might grant money for a pilot of AI in journalism. Also, philanthropic entities in Bangladesh might donate to a platform that promotes national pride and mental well-being through good news. Government or corporate social responsibility (CSR) funds could be a source, though taking government money might complicate perceptions of independence.
In the long run, a diverse revenue mix is healthiest: some income from the community (votes/subscriptions), some from advertisers or sponsors, and perhaps ancillary revenue like merchandise. It’s not far-fetched that a popular positive-news brand could sell merchandise – e.g., t-shirts with inspirational slogans, or publish an annual book of “Bangladesh’s Best Good News 20XX” for sale. Good News Network has a store for merchandise and has published books compiling their stories (About GNN - Good News Network), which suggests additional income streams once a brand is established.
One must also consider the economic context of the audience. Bangladesh’s per capita income is lower than Western countries, so relying on user payments has to be calibrated. $1 might seem small, but if someone voted daily that’s $30 a month, which is not negligible. The platform might keep the voting completely optional (it is by design – only those who care to influence the definition will pay). Most readers can consume content for free, which helps grow audience and fulfill the mission of spreading positivity, while a smaller segment essentially bankrolls the operation. This is similar to the model The Guardian uses: no paywall, but appeals to a minority of readers to contribute voluntarily. That model has seen some success globally, and in our case the contributions are “gamified” as votes, which could actually motivate people more than a simple donation.
In conclusion, the concept can be economically viable if it capitalizes on its strengths: an engaged community (for crowdfunding) and highly brand-safe content (for advertisers). A combination of micro-payments from users, advertising, sponsorships, and possibly subscriptions can be blended. Early on, careful budgeting will be needed and perhaps external seed funding, but if the platform gains traction, it has multiple paths to sustainability. Additionally, by automating content production, it avoids the heavy salary overhead of traditional newsrooms, meaning it can break even with lower revenue. The focus on positive news might even unlock unique revenue sources (like partnerships with mental health campaigns or educational programs that use the content) that typical news sites wouldn’t have. Flexibility and diversified income will be key to weather the uncertainties as the platform finds its audience.
AI Bias and Moderation Risks
Relying on AI to moderate and run the newspaper introduces significant risks of error and bias. AI systems are only as good as their training data and algorithms, and they can misinterpret or even be manipulated. Several risk areas must be addressed:
- Defining “Positive” Is Subjective: Training an AI to recognize “positive news about Bangladesh” is not straightforward. What if a story has a positive outcome but stems from a negative event? For example, a report on how communities rebuilt after a flood could be very uplifting (resilience, aid given, etc.), but an AI might latch onto words like “flood” or “damage” and flag it as negative. Without nuanced understanding, the AI could mistakenly reject genuinely positive stories that contain some negative context. This is a false negative scenario in moderation. It will be crucial to fine-tune the AI to distinguish context – something current AI struggles with. As one analysis of AI in news noted, these systems struggle with subtleties, irony, or euphemisms (Benefits & Challenges of Using AI for Content Moderation). A sarcastic submission like “Great, another power outage – we get to have candlelight dinners!” might confuse AI sentiment analysis which might read “great” and “candlelight dinners” as positive and let a clearly negative news (power outage) slip through. Conversely, a story praising a person “for bravely fighting corruption” might be blocked because the word “corruption” is present, even though the story’s angle is positive. This highlights the challenge of context that an AI must overcome.
- Bias in AI Training: AI models can inherit biases from their training data (What Percentage of News Articles Are AI Generated?). If the model is trained on data that has certain biases (say, more positive stories about urban areas than rural, or biases towards majority groups), it may systematically favor some types of content. For instance, the AI might rate stories about major cities or certain industries as “positive” more often because the training data had many celebratory news from those domains, while overlooking positive news from marginalized groups or remote regions. AI bias could thus lead to unequal representation. Another aspect is if the AI’s dataset includes state media or propaganda that labels only pro-government news as positive – the AI might learn to mimic that, effectively sidelining community-level positive news that isn’t state-sanctioned. The Chekkee analysis of AI moderation warns that if trained on biased datasets, AI will “inadvertently learn and perpetuate those biases,” even associating certain words or images with stereotypes (Benefits & Challenges of Using AI for Content Moderation). For example, it might undervalue a positive story about a tribal community if the data had fewer examples of such content, or misclassify an image of a peaceful protest for environmental reform as negative because protests are generally seen as negative in training data, even though the outcome is positive. Regular audits of the AI’s decisions would be needed to catch such biases.
- False Positives (Allowing the Wrong Content): On the flip side of false negatives, the AI might let through content that violates the spirit of “positive news” because it was cleverly phrased. Determined users might attempt to manipulate the AI. For example, someone with an agenda to spread misinformation could couch a fake story in extremely positive language to trick the system. An AI might focus on the positive tone and miss that the factual claim is bogus. We could see a scenario of false good news, like “Bangladesh invents miracle cure for diabetes!” which is untrue but phrased as great news – the AI might publish it if it’s not equipped for fact-checking. Such misinformation could mislead the public and damage the platform’s credibility. Users might also try to slip in political propaganda disguised as positive development. For instance, a partisan could submit “Thanks to [Politician X], 5,000 villagers now have water” – a positive story on the surface, but it might be propaganda or contain false numbers. If the AI isn’t verifying details, it might post it, effectively allowing a propaganda piece. Malicious actors often find ways to jailbreak AI content filters by using synonyms or indirect phrasing (DeepSeek Uncensored: How One Redditor Tricked the AI Into ...). The platform’s adversaries (or internet pranksters) could test and find loopholes in the positivity filter – for instance, using coded language to include negativity that the AI doesn’t recognize. Continuous refinement and possibly a feedback mechanism (users flagging content that slipped through wrongly) will be needed to catch these.
- Lack of Editorial Oversight and Accountability: Fully removing humans from moderation means when the AI makes a mistake, it might go unnoticed until after publication. Traditional newsrooms have editors to catch errors or tone issues, whereas an AI-run system might publish something tone-deaf. As one analysis pointed out, AI-only news outlets lack the human judgment that prevents factual errors or inappropriate content from going live (What Percentage of News Articles Are AI Generated?). There have been high-profile examples: an AI-written article for a major site contained factual errors and had to be retracted, and an automated system falsely reported a public figure’s death when they were alive (What Percentage of News Articles Are AI Generated?). If our AI misinterprets information, similar embarrassing mistakes could occur. For example, if a user submits “former minister acquitted of corruption charges,” the AI might see “acquitted” (a positive outcome for that person) and publish it as positive news – but this could be quite controversial or even seen as whitewashing corruption. Who is accountable for that decision? The AI can’t be “fired” or sued in the way a human editor could. The platform’s owners would bear the brunt, reputationally and legally. Thus, there is a risk of diminished trust: readers might question if stories are accurate knowing they are AI-curated. Ensuring a way to correct mistakes (perhaps an editor can retroactively edit or remove AI-published pieces) is important for accountability (What Percentage of News Articles Are AI Generated?).
- Manipulation of the Voting System: While not an AI flaw per se, the daily democratic definition can be gamed, which in turn affects the AI’s behavior (since it follows the user mandate). If a group of users (or a rival entity) decided to skew the vote, they could pay for votes to push a certain agenda. For example, a group might vote that “positive news” means “news that praises a certain political party” on a given day. The AI would then, following the rule, approve only such content. This effectively hijacks the platform’s neutrality. Since votes cost money, it’s a bit of an economic barrier to manipulation, but for those with deep pockets (or crowd-funded campaigns) it’s not impossible. The platform could wake up one day to find the “definition” voted by a campaign that doesn’t align with its mission (e.g., in a dystopian scenario, if a hate group paid to define positive news in some twisted way). Safeguards might be needed, like the ability to void obviously malicious definitions or having a baseline definition that can’t be completely overridden. Nonetheless, this introduces governance questions – if the platform overrides a democratic vote due to suspected manipulation, some users might lose trust in the process.
- AI Misunderstanding Cultural Nuance: Bangladesh has cultural and religious nuances that AI might not grasp. A story that seems positive to one demographic might be sensitive to another. For example, a story about a new church opening could be positive news for the Christian community, but the AI might not know to handle religious balance and either block it (thinking religious content might “hurt sentiments” due to DSA) or publish it and face backlash from some hardliners. Human editors typically weigh such sensitivities. AI might also not catch satire or local humor. If someone submits a satirical “good news” piece (e.g., “Finally, Dhaka traffic is so bad that people are walking – good for health!” intended as a joke), the AI might publish it earnestly, confusing the audience and possibly upsetting authorities who don’t appreciate satire.
- Bias Reinforcement through Feedback Loops: There is a risk that the AI + user vote system forms a feedback loop that amplifies certain biases. If the regular voters lean, say, very nationalistic, they will define positive news in nationalistic terms repeatedly. The AI will adapt to that, and only those kinds of stories get published. That in turn attracts more of a similar audience and perhaps alienates others. Over time, the content could become one-dimensional. It’s akin to how algorithms on social media create echo chambers. The platform could end up only highlighting government victories, big infrastructure projects, etc., and neglecting the smaller human-scale good news, or vice versa, depending on who dominates the votes. This could skew public perception just as badly as negativity bias would – it’s a positivity bias where only a certain flavor of positivity is seen. Ensuring diversity in what is considered “positive” is important (covering social, economic, environmental, cultural successes, from all regions and communities). That may require intentionally broad training data and perhaps some rules to not repeatedly publish the same theme every day even if voters vote it (some kind of rotation or encouragement of variety).
In light of these risks, several mitigation strategies should be considered. First, maintain a human oversight layer – even if minimal – such as an editor checking content daily, or a volunteer panel that reviews randomly selected stories. Second, implement robust feedback channels: allow users to flag content that slipped through that they think is inappropriate or incorrect. If many flag a story, it can be withdrawn for review. Third, continuously evaluate the AI’s performance. Use test cases to see if the AI is making biased decisions (e.g., feed it sample positive stories across different domains to ensure it approves them uniformly). If biases are found, retrain the model with more balanced data. Fourth, guard the voting against abuse: maybe limit how many days in a row the same number can vote to prevent one person funding a single narrative, or use identity verification for large voters. The platform could also cap how radically the “definition of positive” can swing – keeping some consistency to avoid confusing the AI or users.
Ultimately, no AI moderation will be perfect. Mistakes and biases will occur; the key is how quickly they are corrected and learned from. Transparency can help here: if the AI does err (say publishes a fake good news), publicly acknowledge the error, correct it, and explain if possible. This builds user trust that the system, while automated, is responsibly managed. In summary, AI gives the platform scalability and consistency, but also inherits the well-known issues of AI in media – potential bias, lack of understanding, susceptibility to exploits, and ambiguity in accountability (What Percentage of News Articles Are AI Generated?) (What Percentage of News Articles Are AI Generated?). Addressing these proactively will determine whether the newspaper is seen as a credible source or just an algorithmic curiosity.
Scalability and Potential for Global Expansion
If successful in Bangladesh, this model could be adapted and expanded to other regions or even a global scale. The underlying concept – user-generated positive news moderated by AI – is not country-specific, but its implementation must respect local languages, culture, and laws. Here’s how scalability might work and what challenges/opportunities arise in going global:
Scaling Within Bangladesh: First, even within Bangladesh, scalability means handling growth in content volume and user base. The infrastructure can be scaled by deploying cloud services that auto-scale with load (many global tech services are available locally or regionally to ensure latency is low). As more users join, the AI models might need periodic retraining to encompass new types of content. An interesting aspect is the multilingual nature of Bangladesh (Bangla is primary, but English is used and minority languages exist). The platform could start Bangla-heavy but eventually support other languages (Chittagonian dialect stories, etc.) which would mean training additional models or using multilingual ones. A scalable approach could be to incorporate a translation layer – allowing someone to submit in any language, auto-translating it to a base language for moderation, then translating final content back. This is doable with current AI translation tools, though nuance can be lost.
Adapting to Other Countries: To launch in another country, one would essentially replicate the system with local adjustments. For example, a “Positive News India” could be envisaged, where citizens across India send WhatsApp news of good happenings, and AI filters for only positive Indian news. The WhatsApp-based submission and distribution model would work in many places – particularly in South Asia, Latin America, Africa, and parts of Europe where WhatsApp is a dominant communication tool for communities. (In fact, during crises or elections, WhatsApp is already used to spread both information and misinformation, so a controlled channel for good information might be welcome.) In countries where WhatsApp isn’t as prevalent (e.g., East Asia or the US), the model could switch to other platforms: perhaps a Telegram bot in Russia or a WeChat integration in China (though China’s regulations would be a massive hurdle), or an SMS-based system in places with more basic phones.
Each country’s notion of “positive news” will differ. The democratic voting mechanism could be replicated so that local users define positivity in their context. One nation might lean towards economic success stories, another towards stories of social harmony, depending on cultural values. The AI models would need to be trained on local language data and news context. Fortunately, many NLP models nowadays are multilingual or have local versions (for major languages like Hindi, Spanish, Arabic etc., there are established sentiment models). The technical lift is training/validating them for the task of filtering news. For lesser-resourced languages, expansion might require collecting a new dataset of positive vs negative news examples to fine-tune models – this is a non-trivial but solvable task if the project has international partners.
Legal and Political Considerations Globally: Ironically, the areas where a “only positive news” outlet might thrive (in terms of acceptance) could be places with restricted press freedom, because it doesn’t challenge authorities. However, those same places often have heavy regulation that could impede a citizen-driven platform. For example, in the Middle East, governments might allow a site that only praises the country, but they typically mandate licenses and might want direct oversight. In democratic countries with free press, there’s no legal barrier to such a platform, but public perception is the bigger issue – it could be seen as fluff or propaganda unless positioned carefully (likely as a supplement, not replacement, to hard news).
The Bangladeshi model could serve as a prototype that, if it gains credibility as a positive journalism source, inspires similar initiatives. Perhaps it could even become a global network: “Positive News Network” chapters in different countries, sharing the same AI technology but guided by local user votes. Content from one country’s platform could be shared to another when relevant – for instance, a great environmental success story in Bangladesh might be featured as an inspirational piece on a global positive news feed, promoting cross-pollination of good ideas.
Cultural Tailoring: Scalability must respect local culture. The AI must learn local taboos of what not to publish even if superficially “positive.” For example, a “positive” story about a minority religious festival might be fine in one country but could spark backlash in another if not handled respectfully. This means for each locale, the AI moderation may need custom rules. It might be wise to involve local journalists or advisors to outline what boundaries the AI should keep in mind (this is akin to content policy guidelines that social platforms have, but in this case focusing on positive framing and local sensitivities).
Multi-country Operations: If the project expanded, a central team could maintain the AI infrastructure, while local teams handle community engagement and regulatory compliance. Each country’s platform could generate revenue independently through local ads or sponsors. There might even be a possibility of a global positive news currency or token – though that gets into speculative territory. Simpler: the concept could be franchised or licensed to local operators who know their market.
Global Audience: Another angle is not going country by country, but broadening the content to global good news. There are already global websites (like GNN) doing this, but our model’s differentiator is the crowd-sourced aspect. One could envision an international version where anyone worldwide submits positive news of their locale, and users globally vote on criteria, etc. However, “positive” might lose coherence globally – it’s easier to build community around a country or culture. People are naturally invested in good news about their own communities. So likely, a global rollout would still be segmented by country or region for the content submission and voting, even if the technology backbone is shared.
Scalability of AI: Technically, scaling to multiple countries means scaling AI training and computation. Cloud providers can handle multi-region deployment, and many AI models can be reused with language-specific tweaks. If the model uses a multilingual backbone (like a multilingual BERT), a lot of the core tech can be the same, just fine-tuned on each new language. This is efficient. The WhatsApp interface can remain largely the same – just different phone numbers or chatbots for each region. So the model is quite replicable.
Challenges in Different Environments: In some countries, the user behavior might differ. For example, in countries with higher literacy in digital media, users might expect more interactive features or might be more critical of AI errors. In others, just having a WhatsApp news source might be revolutionary and quickly adopted. The model might face competition from existing local news channels or social media groups that do similar curation manually. It would need to prove its value (speed, breadth, purity of positivity) over those.
Opportunities: A successfully expanded network could share best practices. If the Bangladesh AI learns extremely well to differentiate nuanced positive content, that improvement can be transferred to other locales. Conversely, lessons learned from elsewhere can refine Bangladesh’s system. There could even be an opportunity to partner with international organizations (like the UN or global NGOs) who are interested in promoting success stories – they might support expansion to countries where highlighting progress can help, for example, in achieving Sustainable Development Goals, a positive-news platform could bolster awareness of local achievements.
One must consider that “positive news only” might be met with skepticism in countries with a very polarized or cynical media climate. For global success, it would be important to emphasize that this model is additive to the news environment – it’s providing a focused stream of good news, not trying to hide bad news. In free societies, as long as that’s clear, it can find its niche of readers who want an antidote to negativity. In more controlled societies, it could ironically become one of the few “safe” citizen journalism outlets (since it avoids criticism).
In essence, the concept is technically and operationally scalable to other countries with adjustments for language and law. The appetite for good news is somewhat universal – numerous surveys show people everywhere feel overwhelmed by negative media and would welcome more positive stories (Constructive Journalism | World's Best News) (Constructive Journalism | World's Best News). By empowering local citizens to share those stories, the platform could tap into an underutilized source of content everywhere. If expansion is pursued, a careful pilot in a second country (maybe one with some similarities to Bangladesh’s media context, like another South Asian country) could be a next step, then iterate from there.
Conclusion & Recommendations
Expanding beyond Bangladesh could multiply the impact of this idea, but it should be done incrementally. Ensuring the model works well in one country (Bangladesh) – both technically and in community uptake – is the priority. Then, documentation of that success can be used to pitch the concept in other markets. Forming a global community of “positive news” contributors could even lead to intercultural exchange of uplifting stories. The ultimate vision might be an AI-driven global positive news network where each locale contributes its best news and the world can share a bit of each other’s joy and progress. This may sound idealistic, but the building blocks are in place, and this Bangladesh digital newspaper could be the pioneering prototype that shows the world how it can be done.